Smart LQA is a new level of linguistic quality evaluation
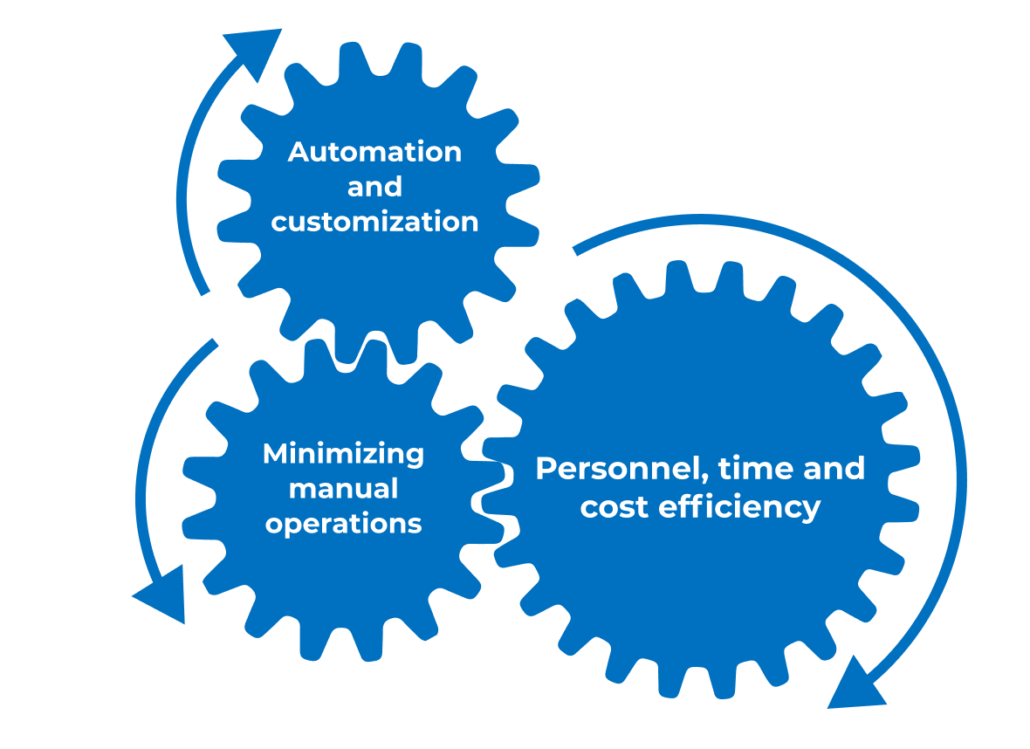
Quality control as a component of quality management is essential to maintain and continuously improve the quality of services provided to the client. This is of paramount importance to our company. Linguistic services constitute the core business process at Janus, so the idea of creating a tool to evaluate the quality of these services has been in development for a long time.
Our objective was to automate this process, reduce the time spent on manual tasks, and streamline the labor-intensive analysis of quality evaluation data and manual launch of linguistic quality assurance tasks. We believe that we have successfully achieved this goal.
Previously, this work was handled outside the information system. The process of launching a linguistic quality evaluation project was based on the expert judgment of production department staff (aside from obvious triggers such as a new client or a new subject area). They would create their own schedules for evaluating vendors across language pairs and subject areas to guarantee the highest level of service quality. However, this type of work is ideally suited to program customization and machine algorithms.
The result is a highly technological innovation. The machine algorithms for data processing and the data itself from completed evaluations essentially mean that we have achieved an AI-driven Linguistic Quality Management system. Another benefit is that quality assurance has moved from a reactive to a proactive process: the Smart LQA system automatically launches linguistic quality evaluation projects at the optimal frequency based on the accumulated data and defined conditions (triggers).
The introduction of triggers or events that prompt the launch of a quality evaluation project is a significant step forward. Previously, quality vector analysis also posed a challenge, as it required identifying whether the trend for a specific client, translator, or language pair was improving or deteriorating. After all, this involved synthesizing disparate information, even for a single client.
For this reason, we decided early on in the design process to store all data in the enterprise information system.
Architecturally, the system is divided into three modules.
Module 1. Triggers
This module oversees the launch of linguistic quality evaluation projects. It consists of a set of flexible triggers or conditions that, when met, require a linguistic quality assessment to be carried out. These conditions may include: the introduction of a new client or language pair, exceeding the maximum time allowed between assessments, the frequency of use of a language pair, or the score received in a previous quality assessment. In addition to direct launch conditions, such as a new client or a new language pair, the AI adjusts the frequency of assessments based on the full set of remaining conditions. This means that the trigger or condition for launching a quality assessment is not fixed, but rather depends on a number of situational factors.
Module 2. Operational module
Unfortunately, it is still not possible to completely eliminate manual work. When assigning tasks to vendors (those who directly perform the linguistic quality evaluation), it is necessary to provide detailed instructions for assessing specific projects, share relevant information for the assessment, maintain an assessment or error log with documented results, and use a form to calculate final scores. All of this is now implemented in a single system. In addition, employees with an academic background in the humanities tend to be less systematic and more creative in their approach to work. This, in turn, can lead to delays when multiple contributors are involved in different stages of the workflow, such as:
- Assessing the quality of linguistic services,
- Familiarizing the vendor with the results,
- Arbitrating any disagreements with the evaluation results,
- Finalizing the score based on the arbitration results.
This could extend the timeframe for completing the workflow and ultimately delay the final results. Essentially, the quality and speed of obtaining evaluation results depended on the level of engagement of the various project participants.
To address this, the process of launching linguistic quality evaluation projects and interacting with project participants has been transformed into a business process within the information system. Each stage now has a task with a deadline, and a notification is sent to the project participant responsible for completing the task. This has helped us systematize the workflow and make deadlines more predictable.
I should note that while AI is making progress in translation evaluation, this is currently limited to a relatively narrow set of language pairs and subject areas. Human involvement in linguistic services and quality evaluation will continue to be necessary for a long time to come, and these humans will need modern tools to work with.
Module 3. Reporting
This is the most important part in terms of data visualization and analysis of linguistic quality evaluation results. Previously, the data extracted from each quality assessment was disparate. The new reporting system can collect data for the required period and in the relevant breakdowns (clients, language pairs, vendors) in a minimum amount of time. It is no longer necessary to compile evaluation results from different files into one large MS Excel file.
And, of course, the Smart LQA project team has several other exciting ideas for future implementation.