Let’s talk about machine translation
Hello, colleagues. Let’s talk about some of the problems and issues associated with machine translation (MT) and its capabilities and achievements.
The first problem that I would like to highlight is that there are some popular languages (for example, Arabic, Korean, Japanese, and Chinese) for which custom machine translation models are seldom used (trained MT – as opposed to untrained or “stock” MT – is MT which has been trained and improved on the basis of a large sample of bilingual texts). For languages where the level of demand might be termed “medium”, things are even worse. In addition, only a small number of companies can provide high-quality MT training for their clients. As for stock (untrained) MT (e.g. Google Translate), again only a small number of companies can provide an acceptable quality.
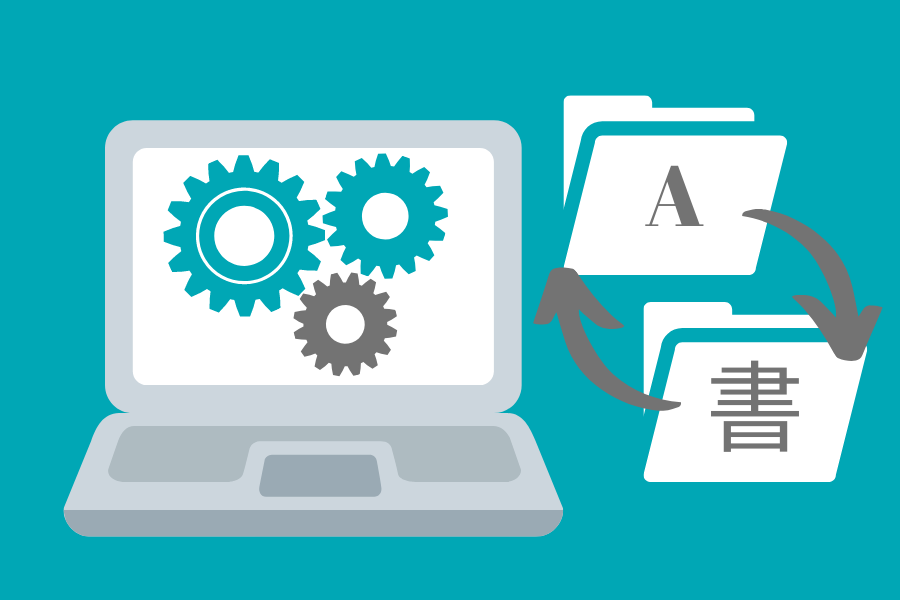
As I understand the situation, the main reason behind poor-quality MT is the fact that most companies do not have a sufficiently large corpora of texts (in other words, a large volume of bilingual texts selected and processed according to certain rules – the set of texts used as the basis for creating MT). This means that a vanishingly small amount of data is used to create the MT models. To date, only a few companies have collected this useful data from a variety of sources over the years, working with professional linguists of an adequately high caliber. It is extremely difficult for newer companies to compete with the large, well-known, experienced companies and to acquire larger corpora of texts.
There are also some companies that can provide their customers with a higher quality of trained MT than the better-known providers, but they are not widely advertised and few people are aware of them. The reputation of MT suffers as a result: people judge the state of MT as a whole by the output from well-known MT providers. However, even this output, which presents a number of disadvantages, can meet the necessary requirements for translation in some circumstances. So a more logical conclusion would be that there is no bad MT, simply cases where a wrongly selected MT approach does not meet certain specific requirements. Few people know about the nuances involved in making the right selection, but they are very familiar to the experts at Janus. Many well-known MT providers have little interest in disseminating this kind of information or educating users in this area, not least since, in doing so, they will inevitably highlight a number of their shortcomings and help users learn about the merits of their competitors. This causes another problem: due to this ignorance, many people have high expectations of MT and expect it to offer a full replacement for human translation, while in fact, the quality of MT very much depends on the subject and type of text involved, as well as on the nuances of the work of a particular MT provider.
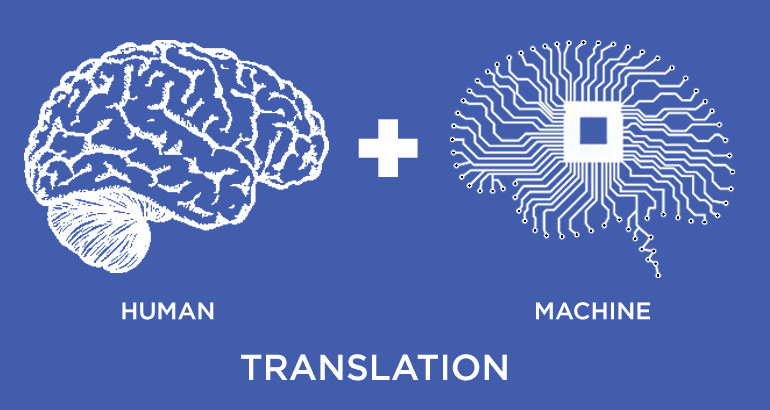
There are languages with which only one (!) MT provider is able to work more or less qualitatively, but this does not prevent other providers from successfully advertising and selling their services. For high-quality translation, good MT models (representing, broadly, the technical side of the issue) and large, correctly prepared corpora of text (the linguistic side) are required. One solution to the lack of good corpora can be to use free texts that are not subject to copyright but are in the public domain. Multilingual websites, scientific articles, examples of the use of words and expressions from dictionaries, encyclopedias, and other types of texts might all be suitable for this purpose. This method cannot be used to create a generic engine, but it is possible to train MT models for specific topics and industries. If people could be encouraged to freely exchange texts spanning different genres and styles in different languages, this would help to develop the field of MT in general. Most MT programs are not comparable to the products of large, well-known providers. Multilingual texts can also be created by technical writers (the main task of a technical writer is to write documents that meet certain requirements). Our company can prepare and provide you with MT produced using MT models created for specific topics.
Another problem is that insufficient studies have been conducted for many languages, including some that are in high demand. However, one of the reasons that few studies and articles are published is that there are few good providers, and among those that are well-known, there are variations in quality. Providers do not offer the same high quality of MT for all languages.
The next problem is the lack of reliable information in MT reports compiled by large, well-known companies operating in the field. MT quality indicators may be underestimated or overestimated for commercial reasons. Our company can provide you with a reliable report on the quality of the text you need translated with the help of various MT providers. To evaluate the quality of MT, we use various methods, including automatic technical evaluation and human evaluation. The technical evaluation compares the characters of the MT with MT text after editing to calculate quality based on the extent to which the MT output differs from the edited version. The MT quality is expressed as a percentage. A competent, correct translation produced by a person from scratch or MT edited in accordance with the customer’s requirements is the 100% benchmark. The human evaluation takes into account the style and similar requirements for translation, as well as other nuances for which quality control cannot be automated.